Deep learning, big data, IoT and blockchain are individually very important research topics of today’s technology, and their combination has the potential to generate additional synergy. Such synergy could enable decentralized and intelligent automated applications to achieve safety, and security and optimize performance and economy. These technologies -Deep learning, big data, IoT, and blockchain all rely on infrastructure capabilities in computing and communications that are increasingly decentralized. Edge computing deployments and architectures are commencing with 5G and are expected to accelerate in 6G. Existing application domains like healthcare and finance are starting to explore the integration of these technologies. Newly emerging application areas such as the metaverse may well require native support of decentralized deep learning to achieve their potential. But the path of new technology development is never smooth. New challenges have been identified and additional architectural frameworks have been developed to overcome some of these issues. Decentralizing deep learning enables increased scale for AI implementations, but also enables improvements in privacy and trustworthiness. The plethora of literature emerging on decentralized deep learning prompts the need for rationale criteria to support design decisions for implementation to utilize decentralized deep learning
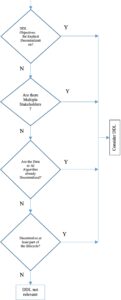
Why Decentralize Deep Learning
Decentralization via blockchains and AI via deep learning are major research and develop trends in modern technology that converge in DDL. The excitement of researchers and technologies for new technologies have led to cycles of unrealistic expectations. The development of suitable criteria to provide a rationale for why DDL should be considered in the designing solutions for commercial implementation should help reduce unrealistic applications. Fig. 1 provides an approach to synthesizing such rationale criteria. This is derived from the discussion in section III to formulate some preliminary questions to help resolve the applicability of DDL as a design solution. Additional criteria could then be developed to extend design decisions further e.g. into selecting particular blockchain or DL algorithms and features, or based on data availability or quality.
The initial enquiry asks whether the objectives of the proposed DDL have an explicit requirement for decentralization. For example, the proposed DDL might be intended for application in an improved type of blockchain which is inherently decentralized.
The second inquiry asks whether there are multiple stakeholders. An internal implementation within a single stakeholder is less likely to require decentralization. Multiple stakeholder collaborating in a more likely to benefit from a trusted decentralized implementation.
The third inquiry asks whether the data or AI algorithm are already decentralized. Where a portion of the design prerequisites are already decentralized, a DDL may be an efficient solution. Where none of the design prerequisites are already decentralized may require a significant transformation of existing processes, implying greater implementation costs.
The fourth inquiry asks whether decentralization is required for at least part of the life cycle. If the decentralization can be constrained to a reduced portion of the lifecycle, this may reduce the implementation costs. Conversely if there is no part of the lifecycle where decentralization makes sense, then DDL does not seem a relevant design solution.
For more information on this topic refer to Wright, S. A. (2023). Why Decentralize Deep Learning? In Workshop on: Decentralized Deep Learning: New Trends and Advanced Technologies (DDL2023) held in conjunction with 2023 IEEE 15th International Symposium on Autonomous Decentralized System (ISADS) (pp. 1-6). IEEE.