Machine learning (ML) is a branch of artificial intelligence that enables computers to learn from data and make predictions or decisions. However, ML can also raise ethical issues and challenges that affect individuals and society. Ethical responsibilities lie with the human stakeholders associated with implementing and adopting ML.
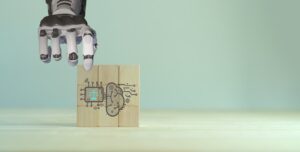
Ethical Responsibilities in ML
Here are some of the types of entities that bear ethical responsibilities associated with the adoption of ML technologies:
– ML developers: ML developers are the people who design, implement, and test ML models and systems. They have an ethical responsibility to ensure that their models are accurate, reliable, transparent, and fair, and that they do not cause harm or discrimination to others. They also have a responsibility to document and communicate their methods, assumptions, limitations, and outcomes of their models to users and stakeholders.
– ML users: ML users are the people who interact with or benefit from ML models and systems. They have an ethical responsibility to use ML in a responsible and informed manner, and to respect the rights and interests of others who may be affected by their actions. They also have a responsibility to provide feedback and report any errors or biases they encounter in ML systems. Some users of ML may have additional professional ethical constraints impacting their use of ML.
– ML organizations: ML organizations are the entities that develop, deploy, or provide ML models and systems. They have an ethical responsibility to ensure that their ML products and services are aligned with their mission, vision, and values, and that they do not harm or exploit their customers, employees, partners, or society at large. They also have a responsibility to monitor, audit, and evaluate their ML systems for performance, quality, and fairness, and to address any issues or risks that arise.
– ML regulators: ML regulators are the entities that oversee or govern the use of ML models and systems. They have an ethical responsibility to ensure that ML complies with legal and ethical standards and principles, and that it protects the rights and interests of individuals and society. They also have a responsibility to establish clear and consistent rules and guidelines for ML development and deployment, and to enforce them effectively.
– ML researchers: ML researchers are the people who conduct scientific or academic studies on ML models and systems. They have an ethical responsibility to ensure that their research is rigorous, valid, reliable, and transparent, and that it contributes to the advancement of knowledge and human well-being. They also have a responsibility to respect the privacy and dignity of their research subjects or participants, and to disclose any conflicts of interest or potential harms or benefits of their research.
– ML educators: ML educators are the people who teach or train others on ML models and systems. They have an ethical responsibility to ensure that their education is accurate, comprehensive, and accessible, and that it fosters critical thinking and ethical awareness among their students or trainees. They also have a responsibility to promote diversity and inclusion in ML education, and to encourage responsible and informed use of ML among their students or trainees.
– ML communities: ML communities are the groups of people who share a common interest or goal related to ML models and systems. They have an ethical responsibility to foster a culture of collaboration, innovation, and excellence in ML development and use. They also have a responsibility to engage with other stakeholders and communities on ML issues and challenges, and to advocate for ethical values and principles in ML.
– ML beneficiaries: ML beneficiaries are the people who receive positive outcomes or impacts from ML models and systems. They have an ethical responsibility to acknowledge the sources and contributions of ML to their well-being or success. They also have a responsibility to share the benefits of ML with others who may not have access or opportunity to use it.
– ML victims: ML victims are the people who suffer negative outcomes or impacts from ML models and systems. They have an ethical responsibility to seek justice or redress for the harms or injustices they experience due to ML. They also have a responsibility to raise awareness and voice their concerns about the issues or challenges they face due to ML.
– ML critics: ML critics are the people who question or challenge the assumptions, methods, outcomes, or implications of ML models and systems. They have an ethical responsibility to provide constructive criticism and alternative perspectives on ML development and use. They also have a responsibility to support evidence-based arguments and respectful dialogue on ML issues and challenges.
Are you a technical, business or legal professional who works with technology adoption? Do you want to learn how to apply ethical frameworks and principles to your technology work and decision-making, understand the legal implications and challenges of new technologies and old laws, and navigate the complex and dynamic environment of technology innovation and regulation? If so, you need to check out this new book: Ethics, Law and Technology: Navigating Technology Adoption Challenges. This book is a practical guide for professionals who want to learn from the experts and stay updated in this fast-changing and exciting field.