Fairness is a moral or ethical concept that involves giving each person what he or she deserves or what is appropriate for the situation. Fairness can be applied to individual actions, interpersonal relations, social institutions, and public policies. Fairness can also be understood as a virtue that guides one’s conduct and character. Law is a legal concept that involves the rules and principles that govern the behavior of individuals and groups within a society. Law can also be understood as a system of authority and enforcement that regulates social order and justice.
Fairness and law are related but distinct concepts. Fairness can be seen as a moral foundation or justification for law, as well as a criterion or standard for evaluating law. Law can be seen as a formal expression or implementation of fairness, as well as a means or instrument for achieving fairness. However, fairness and law can also diverge or conflict in some cases. Fairness can be subjective or relative, depending on one’s perspective, values, or interests. Law can be objective or absolute, depending on its source, validity, or universality. Fairness can be flexible or adaptable, depending on the context, circumstances, or consequences. Law can be rigid or fixed, depending on its form, content, or application.
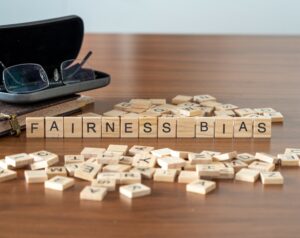
ML Fairness
Fairness as an ethical concept and fairness as a legal concept are not identical or interchangeable. They can complement or support each other, but they can also differ or oppose each other. A fair law is one that is consistent with the ethical principles and values of fairness. A fair action is one that is in accordance with the legal rules and norms of fairness. But a law may not be fair if it violates the ethical rights or interests of some people. And an action may not be fair if it disregards the legal duties or obligations of others.
Machine Learning (ML) technology is a branch of artificial intelligence that enables computers to learn from data and make predictions or decisions. However, ML can also produce unethical results, unfair or biased outcomes that discriminate against certain groups or individuals based on their characteristics, such as race, gender, age, disability, or sexual orientation. Here are some of the issues with fairness resulting from the adoption of ML technology:
- Data bias: ML models depend on the quality and quantity of the data they are trained on. If the data is skewed, incomplete, or inaccurate, it can introduce bias into the model and affect its performance and fairness. For example, if a facial recognition system is trained on mostly white faces, it may not recognize faces of other races accurately.
- Algorithmic bias: ML models are designed and implemented by humans, who may have their own conscious or unconscious biases that influence the choice of features, algorithms, parameters, and evaluation metrics. For example, if a credit scoring system uses gender as a feature, it may discriminate against women who have lower incomes or less credit history than men.
- Predictive bias: ML models make predictions or decisions based on the patterns they learn from the data. However, these patterns may not reflect the true causal relationships or the underlying distribution of the data, leading to errors or inaccuracies that affect some groups more than others. For example, if a recidivism prediction system uses past arrests as a proxy for criminal behavior, it may overestimate the risk of reoffending for minorities who are more likely to be arrested due to systemic racism.
- Feedback loop: ML models can influence the behavior of users or systems that interact with them, creating a feedback loop that reinforces or amplifies the existing biases in the data or the model. For example, if a hiring system recommends candidates based on their similarity to previous hires, it may exclude qualified candidates from underrepresented groups and reduce their chances of applying or being hired in the future.
- Lack of transparency: ML models are often complex and opaque, making it difficult to understand how they work and why they produce certain outcomes. This can limit the ability of users, regulators, or auditors to monitor, explain, or challenge the model’s decisions and hold it accountable for its fairness and accuracy. For example, if a loan application is rejected by a ML system, the applicant may not know the reason or have an opportunity to appeal.
- Lack of interpretability: ML models are often based on statistical correlations rather than causal explanations, making it hard to interpret their results and implications. This can lead to confusion, misunderstanding, or misuse of the model’s outputs and affect the trust and acceptance of users and stakeholders. For example, if a health diagnosis system predicts a high risk of a disease for a patient, the doctor may not know how to communicate or justify this result to the patient or prescribe an appropriate treatment.
- Lack of representation: ML models are often developed and deployed by a small group of experts who may not represent the diversity and needs of the users and beneficiaries of the technology. This can result in a mismatch between the model’s assumptions and objectives and the real-world contexts and scenarios where it is applied. For example, if a speech recognition system is developed by English speakers only, it may not recognize accents or dialects of other languages or cultures.
- Lack of participation: ML models are often imposed on users or communities without their consent or involvement in the design and evaluation process. This can undermine their autonomy, dignity, and agency and expose them to potential harms or risks. For example, if a social welfare system uses ML to assess eligibility for benefits, it may violate the privacy and rights of applicants who have no say in how their data is collected or used.
- Lack of regulation: ML models are often subject to little or no legal or ethical oversight or governance that can ensure their compliance with standards and principles of fairness and justice. This can create loopholes or gaps that allow for abuse or exploitation of the technology by malicious actors or unintended consequences. For example, if a political campaign uses ML to target voters with personalized messages, it may manipulate their opinions or preferences without their awareness or consent.
- Lack of education: ML models are often used by people who have limited knowledge or skills in ML or its ethical implications. This can impair their ability to critically evaluate and responsibly use the technology and its outcomes. For example, if a teacher uses ML to grade students’ assignments, they may not be able to detect errors or biases in the model’s feedback or provide adequate guidance and support to students.
Are you a technical, business or legal professional who works with technology adoption? Do you want to learn how to apply ethical frameworks and principles to your technology work and decision-making, understand the legal implications and challenges of new technologies and old laws, and navigate the complex and dynamic environment of technology innovation and regulation? If so, you need to check out this new book: Ethics, Law and Technology: Navigating Technology Adoption Challenges. This book is a practical guide for professionals who want to learn from the experts and stay updated in this fast-changing and exciting field.